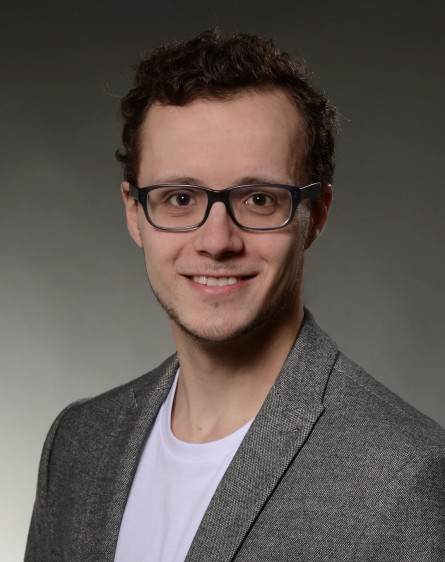
Dear Hannes, what did you do before the CRC, there is not too much about you on the internet.
If you google me, you find "Hannes Salzmann, sand in the gearbox of the capital": Me as a musician at the 1st of May demonstration in Braunschweig. I earned money with music - guitar and singing - as a part-time job. Professionally, I studied in Göttingen. In fact, I started studying information systems technology in Braunschweig. But in the first semester I realised that engineering maths wasn't for me, so I moved to Göttingen to study political science and economics. I then did a Master's degree in political science, focusing on democracy and political party research and on quantitative methods, including supervised and unsupervised machine learning, text as data and quantitative text analysis.
That fits in very well with what is required here at the CRC.
Yes, when I saw the job advertisement, I thought: Wow, that fits like a glove! I was lucky enough to actually get the job. Especially since I had only moved to Bremen with my partner a year ago.
What made you decide to move to Bremen without a job?
My partner and I had studied in different cities. During the pandemic we thought we could study from anywhere. We wanted to go to the north, Hamburg was too big for us - hence: Bremen. I wrote my Master's thesis here and finished in January.
January 2022? That was perfect timing with regard to the position in the CRC.
That was outrageous luck. Especially since I realised during my Master's thesis that I really enjoy research.
What did you examine in your Master's thesis?
Lobbyism. Very exciting, but still under-researched in Germany because the data availability is very poor compared to the USA, for example. In 2013 there was a study at the European level by Heike Klüver. She looked at which factors are decisive for the success of lobbying: how much money does an association have, how many people can it mobilise and how much information does it give to politicians? Klüver compared draft legislation and finalised texts and analysed all the statements of lobbying associations. She used the Wordfish algorithm to do this. The algorithm ranks the texts on a scale - for example, when it comes to the expansion of wind power, between the extreme positions a) "As much wind power as technically possible" and b) "No more wind power at all". This gives us, on the basis of the text documents, a spatial distance between actors.
Klüver then assumed that actors who are on the same side of the scale have entered into a lobbying coalition. Then she looked: Which coalition wins? In which direction did the text of the law move in relation to the original draft? Then she calculated a multiple regression with the factors financial resources of the lobby groups, voter support and information flow. Klüver did this for 56 legislative processes. She was able to prove a statistically significant positive correlation between all three variables and the success of lobbying efforts. Money has the highest influence and voter support the lowest, but the differences are minimal.
In my Master's thesis, I wanted to transfer Klüver's approach to Germany. I collected my own data set on energy policy with about 1500 documents. This was extremely time-consuming because in Germany there is no central place for collecting comments on draft legislation and there is also no obligation to publish them.
When I calculated my regression, I found that I could explain 5 percent of the variance between the draft law and the final text of the law - so it wasn't worth it at all in terms of my research interest! I was only able to show that obviously the data basis in Germany is insufficient to carry out such a lobbying analysis.
Looking back, would you have done anything differently?
Yes, I would have extended my analysis system to include the "degree of proximity" as a variable: Those who merely submit a written opinion are quite far away from the decision-making bodies, but those who meet the federal minister in person are likely to have far-reaching influence. I have researched cases where lobbyists even sat on committees - there, too, one can assume a great deal of influence.
Apart from that, I would narrow down the topic more: Energy policy as a whole was too broad, and the text of the law, with over 300 pages, too extensive. As a result, some of the comments referred to sections of the law that had relatively little to do with each other. I should have done topic modelling beforehand to achieve a stronger focus.
However, it was nice that the automated analysis method allowed me to process text data in a quantity that would never have been possible manually.
In your current work at the CRC, you are following up on these experiences and methods: What exactly are you up to?
I'm now working in the information management project: my first task will be to collect and analyse party programmes. We are trying to determine party positions worldwide and measure their impact on social policy. Traditional ways to determine party positions are to interview experts and to analyse party programmes. But this has disadvantages: Experts are not always available for all parties. And party programmes are not objective data, but strategic documents: their purpose is to present the party to the public in a desired way, and they do not always serve to realistically represent a party's goals. Moreover, a party's position can change in the course of a legislative period.
Therefore, I would like to develop a new approach to measuring party positions. My first idea was about policy output. This has the weakness that you can only apply it to governing parties ...
... basically only to parties that are in government alone ...
Correct! You would have to filter out all other factors, coalition partners, veto players, the Bundesrat, etc. That is difficult.
But there is an archive in Germany with all parliamentary debates, including the names and party affiliations of the speakers. I would like to try to automatically extract ideological positions from parliamentary speeches and derive party positions from them. To do this, I would like to delve a little deeper into quantitative text analysis and natural language processing.
What time period are you looking at?
Which period I'm looking at also depends on the type of algorithm I'm going to use. There are several to choose from. I'm glad that we have two computer scientists in the INF project with whom I can talk about such things. Once I know what is technically possible, I can better estimate how many documents I can analyse and how much pre- and post-processing will be necessary.
Will you limit your analysis to one area of social policy?
I think I will not only look at social policy speeches, but also consider other areas. In determining the party position, I would like to move away from the classic division into left and right - I have in mind a double scale with a libertarian vs. authoritarian and a transverse free-market vs. social justice dimension. My work in the CRC could possibly also benefit from such a classification, as a more precise determination of the parties' position could also provide better insight into the corresponding influence on social policy. In this way, I hope to be able to create further positive synergy effects between my dissertation and my project work.
Contact:
Hannes Salzmann